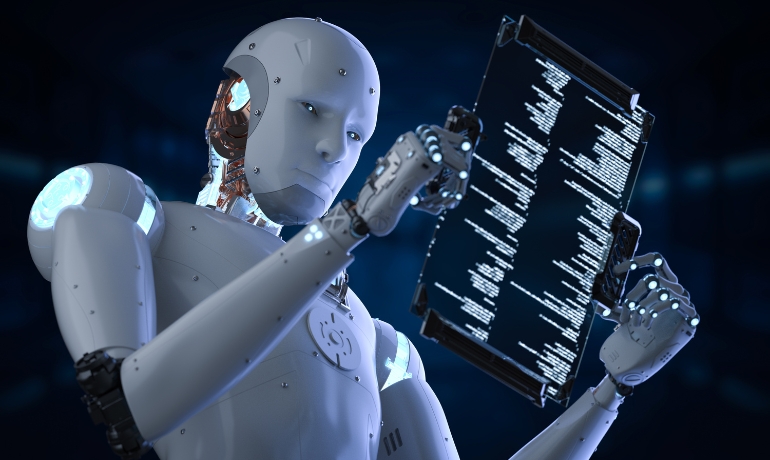
Introduction
As artificial intelligence (AI) becomes increasingly embedded in our daily lives—from decision-making systems in hiring and lending to personalized recommendations and healthcare diagnostics—the need for Responsible AI has never been more crucial. Responsible AI refers to the practice of designing, developing, and deploying AI systems in ways that are ethical, transparent, and aligned with human values. One of the key challenges that Responsible AI can help mitigate is bias and the resulting unfairness in decision-making processes. In this article, we’ll delve into how Responsible AI addresses bias, why it matters, and the broader implications for ethical and equitable AI applications.
Understanding Responsible AI
What Is Responsible AI?
Responsible AI encompasses a set of principles, practices, and policies designed to ensure that AI systems are developed and used in ways that:
- Promote Fairness: Ensure that AI systems do not perpetuate or amplify existing societal biases.
- Enhance Transparency: Provide clear insights into how decisions are made by AI algorithms.
- Protect Privacy: Safeguard sensitive personal data and ensure secure data handling.
- Ensure Accountability: Assign responsibility for AI outcomes and establish frameworks for redress if things go wrong.
- Promote Inclusivity: Involve diverse perspectives in AI development to reflect a wide range of values and needs.
Why Bias Matters in AI
Bias in AI can arise from various sources:
- Data Bias: Training data may reflect historical prejudices, leading to skewed outcomes.
- Algorithmic Bias: The design of AI models might inadvertently favor certain groups or decisions.
- Human Bias: The perspectives and assumptions of developers can influence system behavior.
When left unchecked, bias can lead to unfair treatment of individuals or groups, reinforcing social inequalities and damaging trust in AI systems.
How Responsible AI Mitigates Bias
1. Diverse and Representative Data
- Data Collection: Responsible AI emphasizes the collection of diverse, representative data sets that minimize historical and cultural biases.
- Data Auditing: Regular audits of training data can identify and correct imbalances before they impact AI performance.
2. Fairness-Aware Algorithms
- Algorithm Design: Developers can implement fairness-aware algorithms that adjust decision thresholds to reduce bias.
- Bias Detection Tools: Advanced tools help identify when an AI system’s outcomes are biased, enabling timely interventions.
3. Transparency and Explainability
- Interpretable Models: Responsible AI promotes the use of models that provide clear explanations for their decisions, making it easier to spot biases.
- User-Friendly Reporting: Transparent reporting helps stakeholders understand how decisions are made and on what basis, building trust in the system.
4. Regular Monitoring and Evaluation
- Continuous Feedback: Regular monitoring allows for real-time adjustments to the system as biases are detected.
- Ethical Reviews: Incorporating ethics committees and stakeholder feedback ensures that AI systems are evaluated from multiple perspectives.
5. Accountability and Governance
- Clear Responsibility: Defining accountability for AI decisions ensures that there is a process for addressing and mitigating biased outcomes.
- Regulatory Compliance: Adhering to legal standards and ethical guidelines helps maintain fairness and protect user rights.
Real-World Applications and Benefits
Mitigating Bias in Hiring
AI systems used in recruitment can inadvertently favor certain candidates if they are trained on biased historical hiring data. Responsible AI practices can help ensure that:
- Inclusive Candidate Pools: AI models are trained on data that reflects diversity.
- Fair Screening: Bias detection and correction algorithms help to evaluate candidates based solely on their qualifications.
Ensuring Fair Financial Decisions
In financial services, AI systems influence decisions on loan approvals and credit scoring. By applying Responsible AI principles:
- Equal Opportunities: Bias in credit scoring is reduced, allowing more individuals to access financial services.
- Transparent Decision-Making: Customers receive clear explanations for financial decisions, promoting fairness and trust.
Enhancing Healthcare Outcomes
AI-driven diagnostics and treatment recommendations can be influenced by biases present in medical data. Responsible AI can:
- Improve Accuracy: Ensure that diagnostic tools are trained on diverse patient data.
- Increase Access: Mitigate biases that could otherwise lead to disparities in treatment recommendations.
Conclusion
Responsible AI plays a critical role in mitigating bias—a significant ethical challenge that affects many sectors, from hiring and finance to healthcare and beyond. By prioritizing diverse data, fairness-aware algorithms, transparency, continuous monitoring, and clear accountability, Responsible AI helps create systems that are more equitable, trustworthy, and effective.
While bias is just one of the many issues that Responsible AI aims to address, it stands out as a prime example of how ethical practices in AI development can have a profound, positive impact on society. As technology continues to evolve, embracing Responsible AI principles will be essential for building a future where AI benefits all, regardless of background or circumstance.
Disclaimer: This article is for informational purposes only and reflects current perspectives on Responsible AI and bias mitigation. For specific applications or further research, please refer to academic literature or consult AI ethics experts.
Also Check:
• Can There Be Development Without Overuse? Exploring Sustainable Pathways
• Can the Process of Rusting Be Called Combustion? A Detailed Discussion
• How Can Tireless Striving Achieve Perfection? A Journey of Continuous Improvement
• Can IAS Officer Do Business? Understanding the Rules and Boundaries
1 thought on “What Is Something Responsible AI Can Help Mitigate? Exploring the Impact on Bias and Ethical Challenges”